Maximizing Github ETL Integration

Emma Kessinger
January 03rd , 2021
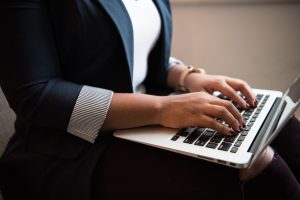
In January 2020, GitHub reportedly had over 40 million users and more than 100 million repositories. This makes GitHub the largest host of source code in the world. Because of that, many companies are seeking a Github ETL integration.
GitHub provides users with popular Git functionalities, such as distributed version control and source code management, as well as its own features like access control and collaboration tools. GitHub provides bug tracking, feature requests, task management, and wikis.
With that much data being shared globally via GitHub, it’s important that collaborators have the ability to organize, understand, and pull insights from it. An ETL process can continuously get all your Github data into a data warehouse where trends can be mined over time. Your Github ETL integration from ETL Robot should capture the following data:
Collaborators
First, a collaborator is someone on the core development team of the project and has commit access to the main repository of the project. By extracting all of that data into a single place, ETL tools let users take a deeper look at who is contributing what to the project. They can identify MVPs by the number of commits, lines added or changed, where those tweaks are made, and whether areas they’re working on are bug-free.
Comments
For any Pull Request, GitHub provides three kinds of comment views: comments on the Pull Request as a whole, comments on a specific line within the Pull Request, and comments on a specific commit within the Pull Request. Collecting comments with our Github ETL integration and categorizing them can help identify who is actively reviewing code and how often.
Additionally, analyzing those discussions can prove useful in all sorts of ways. If comments rapidly grow in a certain area, developers can dedicate their resources to it. Consequently, that can clear up customer support issues stemming from that section of the codebase.
Commits
Commits are code updates developers make to a project. Tracking commits can give a sense of who is contributing the most, and where they are putting their efforts. Our Github ETL integration makes this easy.
Frequency, area, and type of maintenance have all sorts of product development implications. If commits go crazy in one area, is it because of a new feature? Or is an old one acting up? If it’s the latter, it could be worth the company’s while to build a completely new component.
Identifying squeaky wheels is also important for improving the user experience. The key is to compare commits to customer service issues; If hundreds of new commits are found in an area related to common complaints, it’s worth asking if recent commits might be the root cause.
Pull Requests from Your Github ETL Integration
Pull requests are proposed changes to a repository submitted by a developer and accepted or rejected by a repository's collaborators. Thus, these clue other users into the fact that you’ve pushed changes to a certain branch of the software. Collecting data on pulls via ETL can help you see who’s asking for feedback and how often.
Analyzing pull requests helps hold contributors accountable. But more importantly, it ensures that your team is working together effectively.
Pull Request Reviews
Pull request reviews are comments from collaborators on a pull request that approve the changes or request further changes before the pull request is merged.
Also, ETL users can get similar insights from pull request reviews as they can pull requests. How frequently are collaborators providing feedback?
Assignees
Often confused with a reviewer, an assignee is a person dedicated to working on a specific issue or pull request. By retrieving information about assignees through ETL, a company can understand who’s working on what issues over what time frame. As with reviews, that’s critical for understanding workflows and hiring needs.
In conclusion, Github holds all sorts of insights for software developers. But without a Github ETL Integration tool from ETLrobot to continuously load all your data to your data warehouse, it’s tough to get a true understanding of a software’s performance, how well a team is working together, or the quality of committed code. And a high-level view of those things can make a real bottom-line difference.